You signed in with another tab or window. Reload to refresh your session.You signed out in another tab or window. Reload to refresh your session.You switched accounts on another tab or window. Reload to refresh your session.Dismiss alert
adversarial learning 을 사용해서, time-varying data (TVD) 에 대한 spatial supter-resolution (SSR) 을 생성하는 새로운 딥러닝 프레임워크
low-resolution sequence 인 128x128x128 이 input 으로 주어지면,
ouput 으로 high-resolution sequence 인 512x512x512 를 생성하는 네트워크
low-resolution volume sequence 에서 high-resolution volume sequence 를 생성하는 것은 두 가지 과제가 있는데,
high-resolution sequence should maintain good temporal coherence
human perception should be considered in the evaluation of upscaled volumes.
이런 challenges 를 해결하기 위해,
해당 논문에서는
딥러닝 접근 방식을 사용해서, neural network 가 low-resolution volumes 로부터 features 와 그들의 relationships 를 배울 수 있고,
따라서 high-resolution volumes 를 high-wuality 로 생성할 수 있다고 한다.
sparse coding framework 와 image super-resolution technique 에 영감을 받아,
본 논문에서는 SSR-TVD를 제안한다.
a generative model 과
two discriminators 를 갖는
그들의 세 가지 다른 losses를 사용해서,
sequence of volumes 에 대한 spatiotemporally coherent of SSR 을 생성하기 위해
adversarial loss, content loss, feature loss 를 다 고려하는 loss functino 을 최소화해나감으로써 SSR-TVD 를 학습시킴
our approach 의 effectiveness 를 보여주기 위해, several time-varying data sets of different characteristics 를 이용하여 quantitative and qualitative results 를 보여준다.
본 논문의 SSR-TVD 와
widely-used BI 랑
a solution soley based on CNN 를 비교한다.
data, dimage, feature, perception levels 에 대해 더 나은 volume quality 를 달성했다.
third row of Fig.4 shows our SSR-TVD results
original volumes 를 downsampling 해서 low-resolution volumes 를 얻고, inference 할 때 SSR-TVD가 original resolution 으로 volumes back 한다.
The contributions of this paper
GANs 사용
propose novel generative adversarial architecturefor upcaling volume data
comparison of SSR-TVD against BI and CNN (data, image, feature, perception quality metrics)
hyperparameter settings -> analyze impact to performance of SSR-TVD
The text was updated successfully, but these errors were encountered:
peak signal-to-noise ratio (PSNR) : to evaluate quality of synthesized volumes at the data level
structural similarity index (SSIM) : to evalutae the quality of volume rendering images at the image level
besides volume rendering, we also compare against BI in terms of volumetric features, expressed in the form of isosurface
to quantiffy the similarity between two isosurfaces extracted, from the synthesized and GT volumes, we compute their isosurface similarity (IS) at the feature level.
mean option scroe (MOS) : to evaluate how close its rendering image is with respect ot the GT image. -> we recruited ten students and asked them give a five-point score, 1 (loweset perceived quality) ~ 5 (hightest perceived quality) : the mean of these scores is reported as MOS
SSR-TVD 를 BI와 CNN 에 대해서 비교
data level (PSNR)
image level (SSIM)
데이터셋
five jets
vortex
ionization (He+)
ionization (H+)
one variable X of one data se used for training,
another variable Y of the same data set is applited for inference
adversarial learning 을 사용해서, time-varying data (TVD) 에 대한 spatial supter-resolution (SSR) 을 생성하는 새로운 딥러닝 프레임워크
low-resolution sequence 인 128x128x128 이 input 으로 주어지면,
ouput 으로 high-resolution sequence 인 512x512x512 를 생성하는 네트워크
low-resolution volume sequence 에서 high-resolution volume sequence 를 생성하는 것은 두 가지 과제가 있는데,
high-resolution sequence should maintain good temporal coherence
human perception should be considered in the evaluation of upscaled volumes.
이런 challenges 를 해결하기 위해,
해당 논문에서는
딥러닝 접근 방식을 사용해서, neural network 가 low-resolution volumes 로부터 features 와 그들의 relationships 를 배울 수 있고,
따라서 high-resolution volumes 를 high-wuality 로 생성할 수 있다고 한다.
sparse coding framework 와 image super-resolution technique 에 영감을 받아,
본 논문에서는 SSR-TVD를 제안한다.
a generative model 과
two discriminators 를 갖는
그들의 세 가지 다른 losses를 사용해서,
sequence of volumes 에 대한 spatiotemporally coherent of SSR 을 생성하기 위해
adversarial loss, content loss, feature loss 를 다 고려하는 loss functino 을 최소화해나감으로써 SSR-TVD 를 학습시킴
our approach 의 effectiveness 를 보여주기 위해, several time-varying data sets of different characteristics 를 이용하여 quantitative and qualitative results 를 보여준다.
본 논문의 SSR-TVD 와
widely-used BI 랑
a solution soley based on CNN 를 비교한다.
data, dimage, feature, perception levels 에 대해 더 나은 volume quality 를 달성했다.
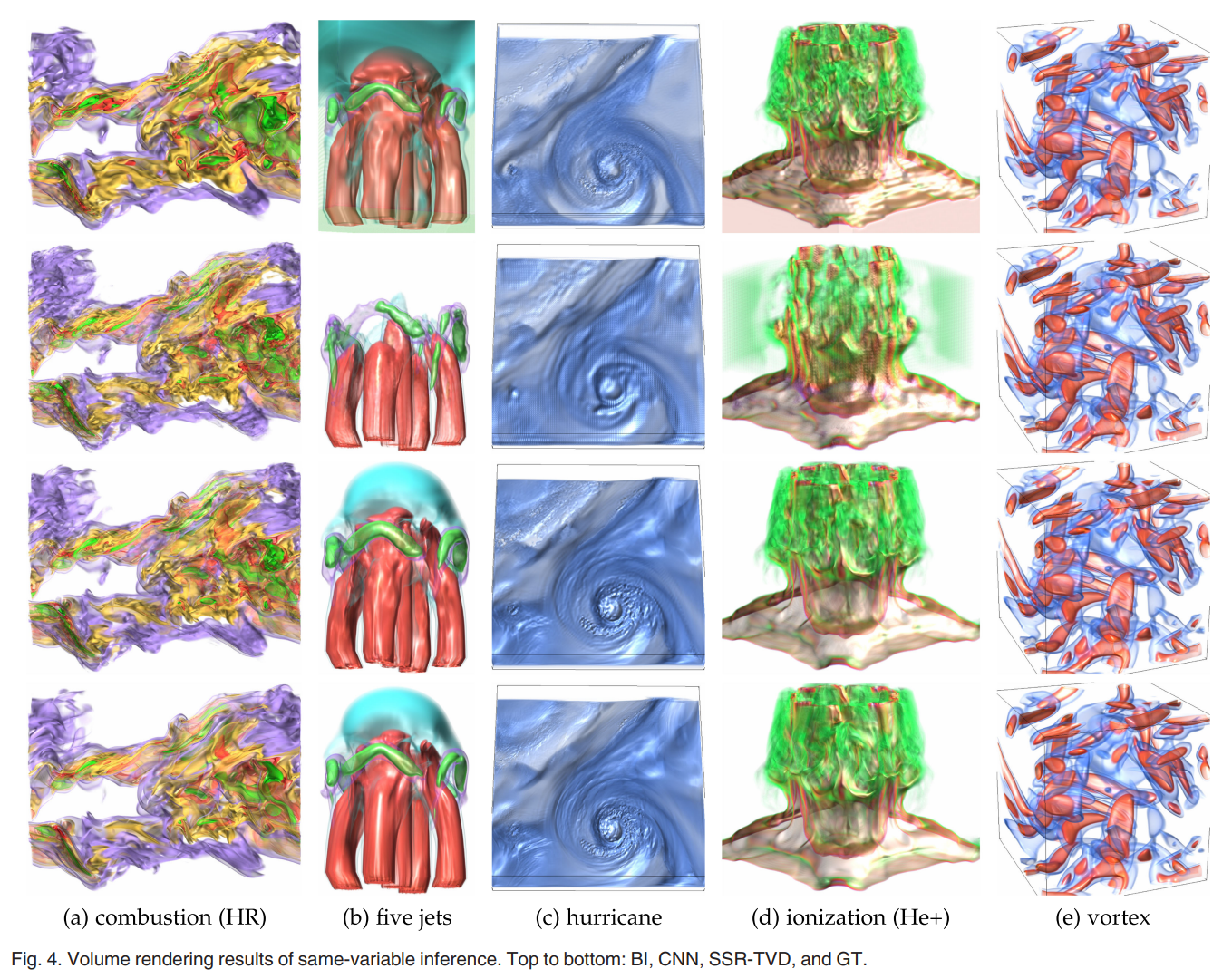
third row of Fig.4 shows our SSR-TVD results
original volumes 를 downsampling 해서 low-resolution volumes 를 얻고, inference 할 때 SSR-TVD가 original resolution 으로 volumes back 한다.
The contributions of this paper
The text was updated successfully, but these errors were encountered: