-
Notifications
You must be signed in to change notification settings - Fork 322
Commit
This commit does not belong to any branch on this repository, and may belong to a fork outside of the repository.
- Loading branch information
Showing
71 changed files
with
526 additions
and
170 deletions.
There are no files selected for viewing
This file contains bidirectional Unicode text that may be interpreted or compiled differently than what appears below. To review, open the file in an editor that reveals hidden Unicode characters.
Learn more about bidirectional Unicode characters
This file contains bidirectional Unicode text that may be interpreted or compiled differently than what appears below. To review, open the file in an editor that reveals hidden Unicode characters.
Learn more about bidirectional Unicode characters
This file contains bidirectional Unicode text that may be interpreted or compiled differently than what appears below. To review, open the file in an editor that reveals hidden Unicode characters.
Learn more about bidirectional Unicode characters
This file contains bidirectional Unicode text that may be interpreted or compiled differently than what appears below. To review, open the file in an editor that reveals hidden Unicode characters.
Learn more about bidirectional Unicode characters
This file contains bidirectional Unicode text that may be interpreted or compiled differently than what appears below. To review, open the file in an editor that reveals hidden Unicode characters.
Learn more about bidirectional Unicode characters
This file contains bidirectional Unicode text that may be interpreted or compiled differently than what appears below. To review, open the file in an editor that reveals hidden Unicode characters.
Learn more about bidirectional Unicode characters
This file contains bidirectional Unicode text that may be interpreted or compiled differently than what appears below. To review, open the file in an editor that reveals hidden Unicode characters.
Learn more about bidirectional Unicode characters
This file contains bidirectional Unicode text that may be interpreted or compiled differently than what appears below. To review, open the file in an editor that reveals hidden Unicode characters.
Learn more about bidirectional Unicode characters
This file contains bidirectional Unicode text that may be interpreted or compiled differently than what appears below. To review, open the file in an editor that reveals hidden Unicode characters.
Learn more about bidirectional Unicode characters
Original file line number | Diff line number | Diff line change |
---|---|---|
@@ -0,0 +1,11 @@ | ||
#!/bin/bash | ||
|
||
set -e | ||
|
||
sed -i s/mirror.centos.org/vault.centos.org/g /etc/yum.repos.d/*.repo | ||
sed -i s/^#.*baseurl=http/baseurl=http/g /etc/yum.repos.d/*.repo | ||
sed -i s/^mirrorlist=http/#mirrorlist=http/g /etc/yum.repos.d/*.repo | ||
|
||
if [[ "$ARCH" = "aarch64" ]]; then | ||
sed -i s/vault.centos.org\\/centos/vault.centos.org\\/altarch/g /etc/yum.repos.d/*.repo | ||
fi |
This file contains bidirectional Unicode text that may be interpreted or compiled differently than what appears below. To review, open the file in an editor that reveals hidden Unicode characters.
Learn more about bidirectional Unicode characters
This file contains bidirectional Unicode text that may be interpreted or compiled differently than what appears below. To review, open the file in an editor that reveals hidden Unicode characters.
Learn more about bidirectional Unicode characters
Original file line number | Diff line number | Diff line change |
---|---|---|
@@ -0,0 +1,56 @@ | ||
# OpenMLDB v0.9.0 Release: Major Upgrade in SQL Capabilities Covering the Entire Feature Servicing Process | ||
|
||
OpenMLDB has just released a new version v0.9.0, including SQL syntax extensions, MySQL protocol compatibility, TiDB storage support, online feature computation, feature signatures, and more. Among these, the most noteworthy features are the MySQL protocol and ANSI SQL compatibility, along with the extended SQL syntax capabilities. | ||
|
||
Firstly, MySQL protocol compatibility allows OpenMLDB users to access OpenMLDB clusters using any MySQL client, not limited to GUI applications like NaviCat or Sequal Ace but also Java JDBC MySQL Driver, Python SQLAlchemy, Go MySQL Driver, and various programming language SDKs. For more information, you can refer to "[**Ultra High-Performance Database OpenM(ysq)LDB: Seamless Compatibility with MySQL Protocol and Multi-Language MySQL Client**](20240322_Openmysqldb.md)". | ||
|
||
Secondly, the new version significantly expands SQL capabilities, especially implementing OpenMLDB’s unique request mode and stored procedure execution within standard SQL syntax. Compared to traditional SQL databases, OpenMLDB covers the entire machine learning process, including offline and online modes. In online mode, users can input sample data, and get feature results through SQL feature extraction. On the contrary, in the past, we needed to deploy SQL as a stored procedure through the `Deploy` command and then perform online feature computation through SDKs or HTTP interfaces. The new version adds `SELECT CONFIG` and `CALL` statements, allowing users to directly specify request mode and sample data in SQL to compute feature results, as shown below: | ||
|
||
``` | ||
-- Execute online request mode query for action (10, "foo", timestamp(4000)) | ||
SELECT id, count(val) over (partition by id order by ts rows between 10 preceding and current row) | ||
FROM t1 | ||
CONFIG (execute_mode = 'online', values = (10, "foo", timestamp(4000))) | ||
``` | ||
You can also use the ANSI SQL `CALL` statement to invoke stored procedures with sample rows as parameters, as shown below: | ||
|
||
``` | ||
-- Execute online request mode query for action (10, "foo", timestamp(4000)) | ||
DEPLOY window_features SELECT id, count(val) over (partition by id order by ts rows between 10 preceding and current row) | ||
FROM t1; | ||
CALL window_features(10, "foo", timestamp(4000)) | ||
``` | ||
For detailed release notes, please refer to: [https://github.com/4paradigm/OpenMLDB/releases/tag/v0.9.0](https://github.com/4paradigm/OpenMLDB/releases/tag/v0.9.0) | ||
|
||
Please feel free to download and explore the latest release. Your feedback is highly valued and appreciated. We encourage you to share your thoughts and suggestions to help us improve and enhance the platform. Thank you for your support! | ||
|
||
## Release Date | ||
|
||
April 25, 2024 | ||
|
||
## Release Note | ||
|
||
[https://github.com/4paradigm/OpenMLDB/releases/tag/v0.9.0](https://github.com/4paradigm/OpenMLDB/releases/tag/v0.9.0) | ||
|
||
## Highlighted Features | ||
|
||
* Added support for the latest version of SQLAlchemy 2, seamlessly integrating with popular Python frameworks such as Pandas and Numpy. | ||
|
||
* Expanded support for more data backends, integrating TiDB’s distributed file storage capability with OpenMLDB’s high-performance in-memory feature computation capability. | ||
|
||
* Enhanced ANSI SQL support, fixed `first_value` semantics, supported `MAP` type and feature signatures, and added offline mode support for `INSERT` statements. | ||
|
||
* Added support for MySQL protocol, allowing access to OpenMLDB clusters using MySQL clients like NaviCat, Sequal Ace, and various MySQL SDKs for programming languages. | ||
|
||
* Extended SQL syntax support, enabling online feature computation directly through `SELECT CONFIG` or `CALL` statements. | ||
|
||
-------------------------------------------------------------------------------------------------------------- | ||
|
||
**For more information on OpenMLDB:** | ||
* Official website: [https://openmldb.ai/](https://openmldb.ai/) | ||
* GitHub: [https://github.com/4paradigm/OpenMLDB](https://github.com/4paradigm/OpenMLDB) | ||
* Documentation: [https://openmldb.ai/docs/en/](https://openmldb.ai/docs/en/) | ||
* Join us on [**Slack**](https://join.slack.com/t/openmldb/shared_invite/zt-ozu3llie-K~hn9Ss1GZcFW2~K_L5sMg)! | ||
|
||
> _This post is a re-post from [OpenMLDB Blogs](https://openmldb.medium.com/)._ |
108 changes: 108 additions & 0 deletions
108
docs/en/blog_post/20240523_OpenmldbFeatureSignatures.md
This file contains bidirectional Unicode text that may be interpreted or compiled differently than what appears below. To review, open the file in an editor that reveals hidden Unicode characters.
Learn more about bidirectional Unicode characters
Original file line number | Diff line number | Diff line change |
---|---|---|
@@ -0,0 +1,108 @@ | ||
# Introducing OpenMLDB’s New Feature: Feature Signatures — Enabling Complete Feature Engineering with SQL | ||
|
||
## Background | ||
|
||
Rewinding to 2020, the Feature Engine team of Fourth Paradigm submitted and passed an invention patent titled “[Data Processing Method, Device, Electronic Equipment, and Storage Medium Based on SQL](https://patents.google.com/patent/CN111752967A)”. This patent innovatively combines the SQL data processing language with machine learning feature signatures, greatly expanding the functional boundaries of SQL statements. | ||
|
||
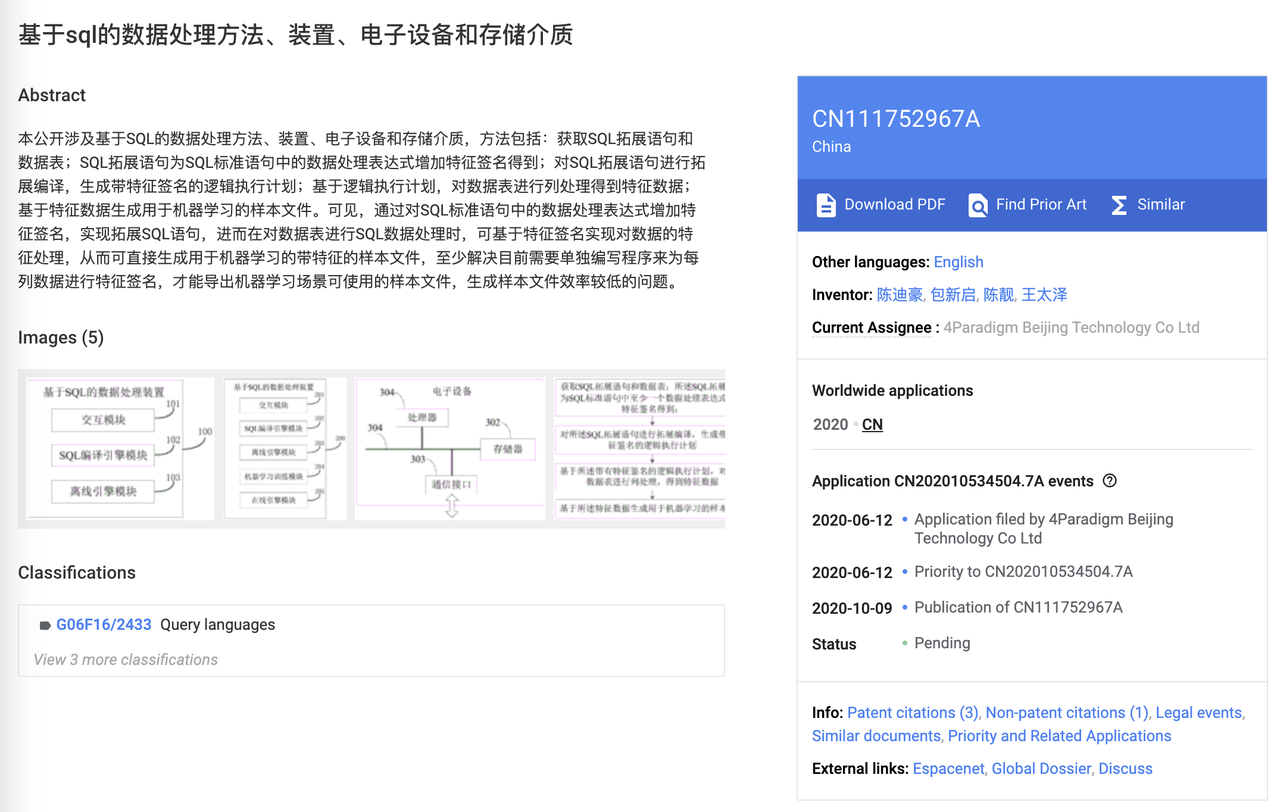 | ||
|
||
At that time, no SQL database or OLAP engine on the market supported this syntax, and even on Fourth Paradigm’s machine learning platform, the feature signature function could only be implemented using a custom DSL (Domain-Specific Language). | ||
|
||
Finally, in version v0.9.0, OpenMLDB introduced the feature signature function, supporting sample output in formats such as CSV and LIBSVM. This allows direct integration with machine learning training or prediction while ensuring consistency between offline and online environments. | ||
|
||
## Feature Signatures and Label Signatures | ||
|
||
The feature signature function in OpenMLDB is implemented based on a series of OpenMLDB-customized UDFs (User-Defined Functions) on top of standard SQL. Currently, OpenMLDB supports the following signature functions: | ||
|
||
* `continuous(column)`: Indicates that the column is a continuous feature; the column can be of any numerical type. | ||
|
||
* `discrete(column[, bucket_size])`: Indicates that the column is a discrete feature; the column can be of boolean type, integer type, or date and time type. The optional parameter `bucket_size` sets the number of buckets. If `bucket_size` is not specified, the range of values is the entire range of the int64 type. | ||
|
||
* `binary_label(column)`: Indicates that the column is a binary classification label; the column must be of boolean type. | ||
|
||
* `multiclass_label(column)`: Indicates that the column is a multiclass classification label; the column can be of boolean type or integer type. | ||
|
||
* `regression_label(column)`: Indicates that the column is a regression label; the column can be of any numerical type. | ||
|
||
These functions must be used in conjunction with the sample format functions `csv` or `libsvm` and cannot be used independently. `csv` and `libsvm` can accept any number of parameters, and each parameter needs to be specified using functions like `continuous` to determine how to sign it. OpenMLDB handles null and erroneous data appropriately, retaining the maximum amount of sample information. | ||
|
||
## Usage Example | ||
|
||
First, follow the [quick start](https://openmldb.ai/docs/en/main/tutorial/standalone_use.html) guide to get the image and start the OpenMLDB server and client. | ||
```bash | ||
docker run -it 4pdosc/openmldb:0.9.0 bash | ||
/work/init.sh | ||
/work/openmldb/sbin/openmldb-cli.sh | ||
``` | ||
|
||
Create a database and import data in the OpenMLDB client. | ||
```sql | ||
--OpenMLDB CLI | ||
CREATE DATABASE demo_db; | ||
USE demo_db; | ||
CREATE TABLE t1(id string, vendor_id int, pickup_datetime timestamp, dropoff_datetime timestamp, passenger_count int, pickup_longitude double, pickup_latitude double, dropoff_longitude double, dropoff_latitude double, store_and_fwd_flag string, trip_duration int); | ||
SET @@execute_mode='offline'; | ||
LOAD DATA INFILE '/work/taxi-trip/data/taxi_tour_table_train_simple.snappy.parquet' INTO TABLE t1 options(format='parquet', header=true, mode='append'); | ||
``` | ||
|
||
Use the `SHOW JOBS` command to check the task running status. After the task is successfully executed, perform feature engineering and export the training data in CSV format. | ||
|
||
Currently, OpenMLDB does not support overly long column names, so specifying the column name of the sample as `instance` using `SELECT csv(...)` AS instance is necessary. | ||
|
||
```sql | ||
--OpenMLDB CLI | ||
USE demo_db; | ||
SET @@execute_mode='offline'; | ||
WITH t1 as (SELECT trip_duration, | ||
passenger_count, | ||
sum(pickup_latitude) OVER w AS vendor_sum_pl, | ||
count(vendor_id) OVER w AS vendor_cnt, | ||
FROM t1 | ||
WINDOW w AS (PARTITION BY vendor_id ORDER BY pickup_datetime ROWS_RANGE BETWEEN 1d PRECEDING AND CURRENT ROW)) | ||
SELECT csv( | ||
regression_label(trip_duration), | ||
continuous(passenger_count), | ||
continuous(vendor_sum_pl), | ||
continuous(vendor_cnt), | ||
discrete(vendor_cnt DIV 10)) AS instance | ||
FROM t1 INTO OUTFILE '/tmp/feature_data_csv' OPTIONS(format='csv', header=false, quote=''); | ||
``` | ||
|
||
If LIBSVM format training data is needed, simply change `SELECT csv(...)` to `SELECT libsvm(...)`. Note that the `OPTIONS` should still use the CSV format because the exported data only has one column, which already contains the complete LIBSVM format sample. | ||
|
||
Moreover, the `libsvm` function will start numbering continuous features and discrete features with a known number of buckets from 1. Therefore, specifying the number of buckets ensures that the feature encoding ranges of different columns do not conflict. If the number of buckets for discrete features is not specified, there is a small probability of feature signature conflict in some samples. | ||
|
||
```sql | ||
--OpenMLDB CLI | ||
USE demo_db; | ||
SET @@execute_mode='offline'; | ||
WITH t1 as (SELECT trip_duration, | ||
passenger_count, | ||
sum(pickup_latitude) OVER w AS vendor_sum_pl, | ||
count(vendor_id) OVER w AS vendor_cnt, | ||
FROM t1 | ||
WINDOW w AS (PARTITION BY vendor_id ORDER BY pickup_datetime ROWS_RANGE BETWEEN 1d PRECEDING AND CURRENT ROW)) | ||
SELECT libsvm( | ||
regression_label(trip_duration), | ||
continuous(passenger_count), | ||
continuous(vendor_sum_pl), | ||
continuous(vendor_cnt), | ||
discrete(vendor_cnt DIV 10, 100)) AS instance | ||
FROM t1 INTO OUTFILE '/tmp/feature_data_libsvm' OPTIONS(format='csv', header=false, quote=''); | ||
``` | ||
|
||
## Summary | ||
|
||
By combining SQL with machine learning, feature signatures simplify the data processing workflow, making feature engineering more efficient and consistent. This innovation extends the functional boundaries of SQL, supporting the output of various formats of data samples, directly connecting to machine learning training and prediction, improving data processing flexibility and accuracy, and having significant implications for data science and engineering practices. | ||
|
||
OpenMLDB introduces signature functions to further bridge the gap between feature engineering and machine learning frameworks. By uniformly signing samples with OpenMLDB, offline and online consistency can be improved throughout the entire process, reducing maintenance and change costs. In the future, OpenMLDB will add more signature functions, including one-hot encoding and feature crossing, to make the information in sample feature data more easily utilized by machine learning frameworks. | ||
|
||
-------------------------------------------------------------------------------------------------------------- | ||
|
||
**For more information on OpenMLDB:** | ||
* Official website: [https://openmldb.ai/](https://openmldb.ai/) | ||
* GitHub: [https://github.com/4paradigm/OpenMLDB](https://github.com/4paradigm/OpenMLDB) | ||
* Documentation: [https://openmldb.ai/docs/en/](https://openmldb.ai/docs/en/) | ||
* Join us on [**Slack**](https://join.slack.com/t/openmldb/shared_invite/zt-ozu3llie-K~hn9Ss1GZcFW2~K_L5sMg)! | ||
|
||
> _This post is a re-post from [OpenMLDB Blogs](https://openmldb.medium.com/)._ |
This file contains bidirectional Unicode text that may be interpreted or compiled differently than what appears below. To review, open the file in an editor that reveals hidden Unicode characters.
Learn more about bidirectional Unicode characters
Oops, something went wrong.